
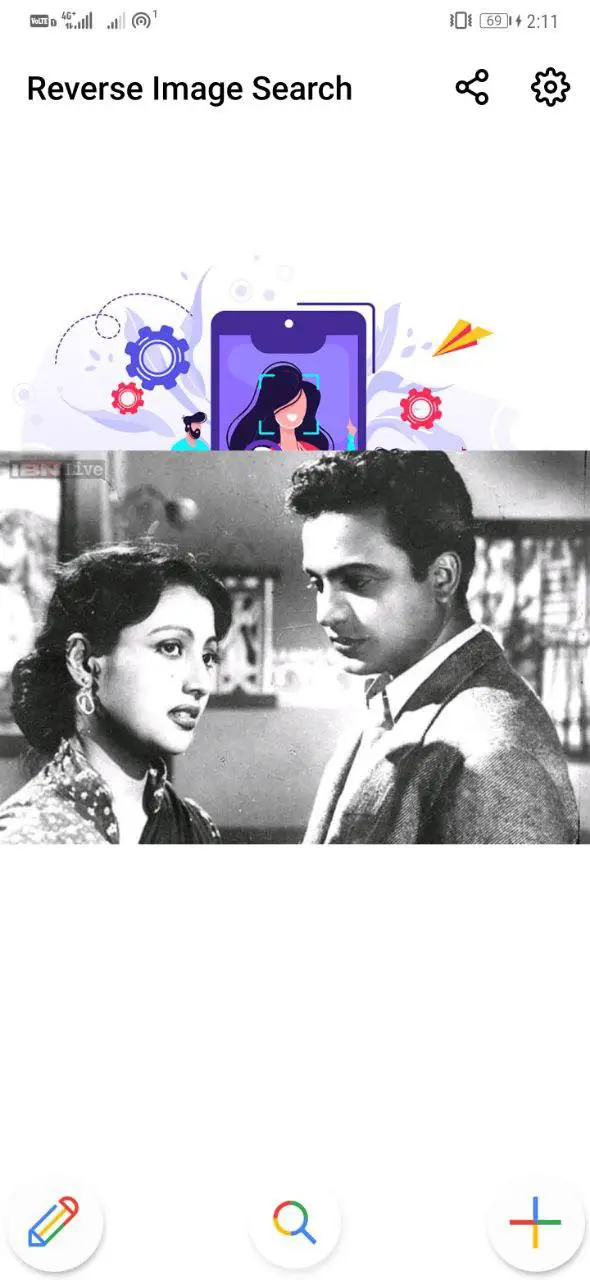
The past decade has seen tremendous progress in image classification using convolutional neural networks, beginning with Krizhevsky et al ’s breakthrough result on the ImageNet challenge in 2012. characteristics of the image itself, such as black-and-white or close-up.overall scene descriptors like outdoors or wedding.specific objects in the image, such as tree or person.Higher scores indicate a higher probability that the image belongs to that category. We build this function using two key developments in machine learning: accurate image classification and word vectors.Īn image classifier reads an image and outputs a scored list of categories that describe its contents. Given this function, when a user does a search we run it on all their images and return those that produce a score above a threshold, sorted by their scores. Here’s a simple way to state the image search problem: find a relevance function that takes a (text) query q and an image j, and returns a relevance score s indicating how well the image matches the query.
